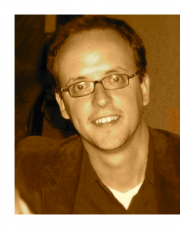
Using Twitter to Detect Micro-Crises in Real-Time
Social media is increasingly used to communicate during major crises. But what about small-scale incidents such as a car crash or fire? These “micro-crises” typically generate a far smaller volume of social media activity during a much shorter period and more bounded geographical area. Detecting these small-scale events thus poses an important challenge for the field of Crisis Computing.
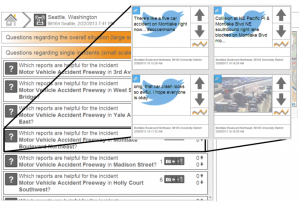
Axel Schulz just published co-authored a paper on this exact challenge. In this study, he and co-authors Petar Ristoski & Heiko Paulheim ”present a solution for a real-time identification of small scale incidents using microblogs,” which uses machine learning—combining text classication and semantic enrichment of microblogs—to increase situational awareness. The study draws on 7.5 million tweets posted in the city centers of Seattle and Memphis during November & December 2012 and February 2013. The authors used the “Seattle Real Time Fire 911 Calls” dataset to identify relevant keywords in the collected tweets. They also used WordNet to “extend this set by adding the direct hyponyms. For instance, the keyword “accident” was extended with ‘collision’, ‘crash’, ‘wreck’, ‘injury’, ‘fatal accident’, and ‘casualty’.”
An evaluation of this combined “text classication” and “semantic enrichment” approach shows that small scale incidents can be identified with an accuracy 89%. A copy of Axel et al.‘s paper is available here (PDF). This is a remarkable level of accuracy given the rare and micro-level nature of the incidents studied.