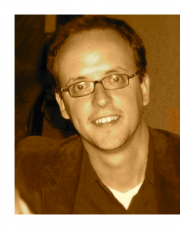
Twitter, Crises and Early Detection: Why “Small Data” Still Matters
My colleagues John Brownstein and Rumi Chunara at Harvard University's HealthMap
I had actually written this blog post on Crimson Hexagon four years ago to explore how the platform could be used for early warning purposes, so I'm thrilled to see this potential realized.
There is a lot that intrigues me about the work that HealthMap and Global Pulse are doing. But one point that really struck me vis-a-vis the former is just how little data was necessary to identify the outbreak. To be sure, not many Haitians are on Twitter and my impression is that most humanitarians have not really taken to Twitter either (I'm not sure about the Haitian Diaspora). This would suggest that accurate, early detection is possible even without Big Data; even with “Small Data” that is neither representative or indeed verified. (Interestingly, Rumi notes that the Haiti dataset is actually larger than datasets typically used for this kind of study).
In related news, a recent peer-reviewed study by the European Commission found that the spatial distribution of crowdsourced text messages (SMS) following the earthquake in Haiti were strongly correlated with building damage. Again, the dataset of text messages was relatively small. And again, this data was neither collected using random sampling (i.e., it was crowdsourced) nor was it verified for accuracy. Yet the analysis of this small dataset still yielded some particularly interesting findings that have important implications for rapid damage detection in post-emergency contexts.
While I'm no expert in econometrics, what these studies suggests to me is that detecting change-over–time is ultimately more critical than having a large-N dataset, let alone one that is obtained via random sampling or even vetted for quality control purposes. That doesn't mean that the latter factors are not important, it simply means that the outcome of the analysis is relatively less sensitive to these specific variables. Changes in the baseline volume/location of tweets on a given topic appears to be strongly correlated with offline dynamics.
What are the implications for crowdsourced crisis maps and disaster response? Could similar statistical analyses be carried out on Crowdmap data, for example? How small can a dataset be and still yield actionable findings like those mentioned in this blog post?