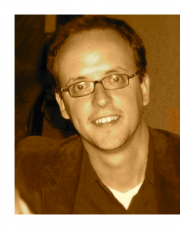
What Percentage of Tweets Generated During a Crisis Are Relevant for Humanitarian Response?
More than half-a-million tweets were generated during the first three days of Hurricane Sandy and well over 400,000 pictures were shared via Instagram. Last year, over one million tweets were generated every five minutes on the day that Japan was struck by a devastating earthquake and tsunami. Humanitarian organi-zations are ill-equipped to manage this volume and velocity of information. In fact, the lack of analysis of this “Big Data” has spawned all kinds of suppositions about the perceived value—or lack thereof—that social media holds for emer-gency response operations. So just what percentage of tweets are relevant for humanitarian response?
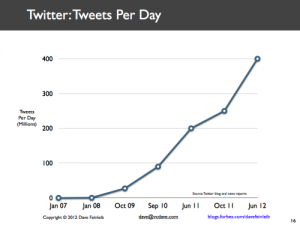
One of the very few rigorous and data-driven studies that addresses this question is Dr. Sarah Vieweg‘s 2012 doctoral dissertation on “Situational Awareness in Mass Emergency: Behavioral and Linguistic Analysis of Disaster Tweets.” After manually analyzing four distinct disaster datasets, Vieweg finds that only 8% to 20% of tweets generated during a crisis provide situational awareness. This implies that the vast majority of tweets generated during a crisis have zero added value vis-à-vis humanitarian response. So critics have good reason to be skeptical about the value of social media for disaster response.
At the same time, however, even if we take Vieweg’s lower bound estimate, 8%, this means that over 40,000 tweets generated during the first 72 hours of Hurricane Sandy may very well have provided increased situational awareness. In the case of Japan, more than 100,000 tweets generated every 5 minutes may have provided additional situational awareness. This volume of relevant infor-mation is much higher and more real-time than the information available to humanitarian responders via traditional channels.
Furthermore, preliminary research by QCRI’s Crisis Computing Team show that 55.8% of 206,764 tweets generated during a major disaster last year were “Informative,” versus 22% that were “Personal” in nature. In addition, 19% of all tweets represented “Eye-Witness” accounts, 17.4% related to information about “Casualty/Damage,” 37.3% related to “Caution/Advice,” while 16.6% related to “Donations/Other Offers.” Incidentally, the tweets were automatically classified using algorithms developed by QCRI. The accuracy rate of these ranged from 75%-81% for the “Informative Classifier,” for example. A hybrid platform could then push those tweets that are inaccurately classified to a micro-tasking platform for manual classification, if need be.
This research at QCRI constitutes the first phase of our work to develop a Twitter Dashboard for the Humanitarian Cluster System, which you can read more about in this blog post. We are in the process of analyzing several other twitter datasets in order to refine our automatic classifiers. I’ll be sure to share our preliminary observations and final analysis via this blog.
Phi Beta Iota: What is really interesting about this is 400 million tweets a day, on its way to 5 billion tweets a day. That is a real-time machine-human processing challenge that could be usefully addressed.